How AI Can Help Achieve Large-Scale Automated Test and Re-Order (LATR) Model for Small Garment Factories
| Feb, 14 , 25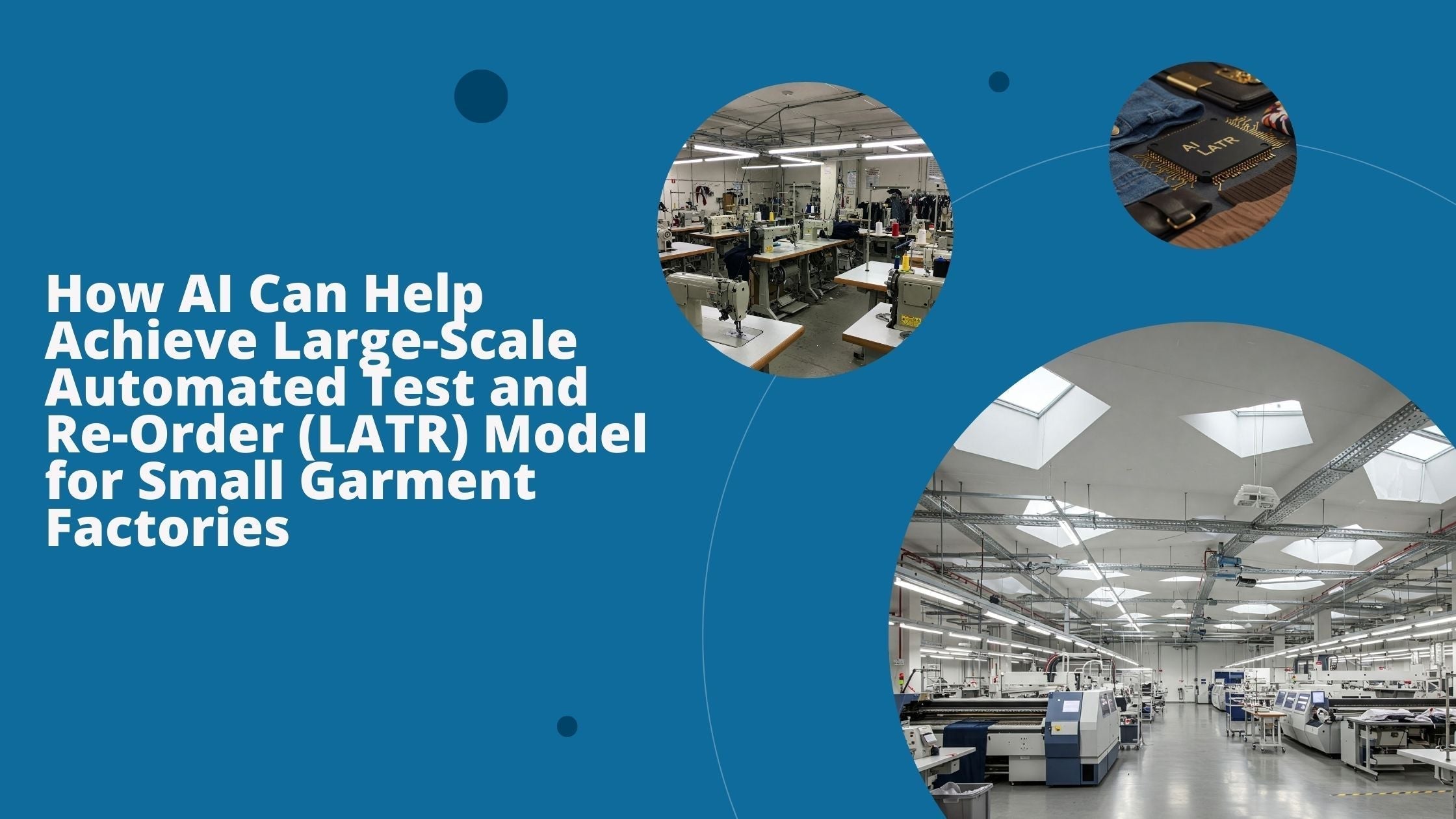
The garment industry has always been dynamic, driven by ever-changing consumer preferences and market trends. The Large-Scale Automated Test and Re-Order (LATR) model has emerged as a powerful approach to keep up with these rapid shifts. The LATR model enables garment factories to minimize waste, reduce lead times, and respond swiftly to market demands by continuously testing new designs in small batches and quickly re-ordering the successful ones.
The LATR model has significantly impacted both established apparel brands and online channels. Traditional brands, which often relied on lengthy production cycles and prominent inventory commitments, have been shaken by the efficiency and agility of the LATR approach. Online channels, already adept at quick turnarounds, have further optimized their operations by integrating the LATR model, allowing them to stay ahead in the fast-paced digital marketplace.
At the heart of the LATR success is the role of Artificial Intelligence (AI). AI technologies offer transformative solutions for implementing and optimizing the LATR model, especially for small garment factories. From demand prediction and automated testing to re-order optimization, AI empowers small garment factories to achieve large-scale efficiencies and compete effectively in the market.
We will explore how AI can help small garment factories leverage the LATR model to enhance their production processes, improve responsiveness, and drive sustainable growth. By understanding the benefits and applications of AI in the LATR model, small garment factories can unlock new opportunities for innovation and success. Let us begin from the basics.

Understanding the LATR Model
Large-Scale Automated Test and Re-Order (LATR) Model
The Large-Scale Automated Test and Re-Order (LATR) model is a revolutionary approach designed to enhance efficiency and responsiveness. It involves producing small batches of new designs, performing retesting, and re-ordering the successful ones in massive quantities. This iterative process allows factories to minimize risks and adapt swiftly to consumer preferences.
Components of the LATR Model:
-
Small Batch Production: Initially, new designs are produced in small quantities. It limits the risk of launching a new product and reduces inventory costs.
-
Market Testing: They introduce these batches to the market. It can be through physical stores, online channels, or both. They can monitor the performance of these designs closely.
-
Data Analysis: Sales data, customer feedback, and other performance metrics are collected and analyzed to determine the success of the new designs.
-
Automated Re-Ordering: Based on the analysis, they can identify and place large orders of successful designs to meet the anticipated demand. This process is automated to ensure quick response times.
-
Iterative Process: The cycle repeats, allowing factories to continuously introduce and test new designs, ensuring they stay current with market trends.
Benefits of the LATR Model
-
Reduced Waste:
-
Minimized Overproduction: By producing in small batches initially, factories and brands avoid the risk of overproducing designs that may not sell well. This approach reduces waste and lowers the environmental impact of unsold inventory.
-
Efficient Resource Utilization: The model ensures that resources such as materials and labor are used efficiently, only committing to massive production once a design success is validated.
-
Improved Efficiency:
-
Streamlined Operations: The LATR model streamlines the production process by focusing on data-driven decisions. Automated re-ordering reduces manual intervention, speeding up the production cycle.
-
Optimized Inventory Management: Factories maintain leaner inventories, as they only stock what is needed based on real-time sales data. This optimization reduces storage costs and enhances cash flow management.
-
Faster Response to Market Trends:
-
Agility and Adaptability: The iterative nature of the LATR model allows factories to quickly adapt to changing consumer preferences and market trends. They can introduce and test new designs, ensuring the product offerings remain relevant.
-
Enhanced Customer Satisfaction: By responding quickly to market demands, factories can offer products that align closely with customer preferences, leading to higher satisfaction and loyalty.
Challenges of Implementing the LATR Model for Small Garment Factories
-
Data Management:
-
Complexity of Data Collection: Collecting and analyzing large volumes of data from various sources can be complex. Small garment factories need robust data management systems to handle sales data, customer feedback, and performance metrics.
-
Accuracy and Reliability: It is crucial for making informed decisions. The tiniest inaccuracies can lead to incorrect conclusions and affect the success.
-
Technology and Infrastructure:
-
Advanced Technology Requirements: Implementing the LATR model requires advanced technology, including AI-driven tools for data analysis, automated re-ordering systems, and real-time inventory management. Small garment factories may face challenges in adopting and integrating these technologies.
-
Initial Investment: The upfront investment in technology and infrastructure can be significant. Small garment factories must assess the cost-benefit ratio and ensure they have the financial resources to support the implementation.
-
Supply Chain Coordination:
-
Supplier Collaboration: Effective implementation of the LATR model requires close collaboration with suppliers to ensure timely delivery of materials and components. Coordinating with multiple suppliers can be challenging when demand fluctuates.
-
Logistics and Distribution: Managing logistics and distribution for small batches and re-orders requires efficient systems. Delays or inefficiencies in the supply chain can disrupt the production cycle and affect responsiveness.
-
Workforce Adaptation:
-
Training and Skill Development: Adopting the LATR model involves changes in production processes and technology use. Workforce training and skill development are essential to ensure that employees can effectively operate new systems and technologies.
-
Change Management: Implementing new models and technologies can face resistance from employees. Effective change management strategies are needed to ensure smooth transitions and acceptance of new processes.

The Role of AI in the LATR Model
Demand Prediction
-
Consumer Demand Analysis:
-
Historical Data Analysis: AI systems can analyze historical sales data, considering seasonal trends, past consumer behavior, and market dynamics to predict future demand. By identifying patterns and correlations in large datasets, AI can forecast which products could be trendy.
-
Real-Time Data Integration: AI can integrate real-time data from various sources, including social media, e-commerce platforms, and market reports. This integration allows for immediate adjustments to predictions based on current events and emerging trends.
-
Trend Identification:
-
Social Media Monitoring: AI can monitor social media platforms to detect emerging trends and consumer preferences. AI identifies which styles, colors, and designs are gaining popularity through hashtags, mentions, and visual content.
-
Sentiment Analysis: Using natural language processing (NLP), AI can perform sentiment analysis on customer reviews and feedback. Understanding consumer sentiment helps to identify positive and negative perceptions of different products and guide design and production decisions.
-
Market Segmentation:
-
Consumer Profiling: AI can segment the market into different consumer groups based on demographics, purchasing behavior, and preferences. This segmentation helps to tailor products to meet the specific needs of various customer segments.
-
Personalized Marketing: AI-driven insights enable marketing strategies, targeting consumers with products that match their preferences. This targeted approach increases the likelihood of successful product launches and higher sales.
Automated Testing
-
Rapid Prototyping:
-
Virtual Design Tools: AI-powered design tools enable rapid prototyping by creating virtual models of new designs. Designers can experiment with different styles, fabrics, and colors without physical samples, speeding up the testing process.
-
3D Simulation: AI can simulate how new designs look and fit different body types. This simulation helps to identify potential design issues early, reducing the need for physical adjustments.
-
Market Testing:
-
Small Batch Production: AI systems can manage the production of small batches of new designs for market testing. By analyzing sales data and customer feedback from these small batches, AI determines the potential success of new products.
-
A/B Testing: AI can conduct A/B testing by launching multiple versions of a design to different consumer segments. By comparing the performance of each version, AI identifies the most appealing design elements and optimizes future production.
-
Feedback Loop:
-
Customer Feedback Analysis: AI can gather and analyze customer feedback from multiple channels, including online reviews, social media comments, and direct feedback. This analysis helps to understand consumer reactions to new designs.
-
Iterative Improvement: Based on feedback, AI recommends design modifications and improvements. This iterative process ensures continuous product improvements to meet consumer expectations before larger-scale production.
Re-Order Optimization
-
Inventory Management:
-
Real-Time Monitoring: AI systems continuously monitor inventory levels and sales data. This real-time monitoring ensures that factories have up-to-date information on stock levels and can make informed re-ordering decisions.
-
Stock Level Optimization: AI can optimize stock levels by predicting demand fluctuations and adjusting re-order quantities accordingly. This optimization prevents overstocking and stockouts, balancing supply and demand.
-
Automated Re-Ordering:
-
Trigger-Based Re-Ordering: AI can set up automated re-ordering triggers based on predefined thresholds. When inventory levels fall below a certain point, AI systems automatically generate re-orders to replenish stock.
-
Supplier Coordination: AI can coordinate with suppliers to ensure timely delivery of materials and components. By optimizing supplier relationships and logistics, AI reduces lead times and ensures smooth production flow.
-
Sales and Demand Forecasting:
-
Predictive Analytics: AI uses predictive analytics to forecast future sales and demand for different products. By analyzing historical data, market trends, and consumer behavior, AI provides accurate predictions for re-order quantities and timing.
-
Seasonal Adjustments: AI can account for seasonal variations in demand, adjusting and re-order the quantities accordingly. This capability ensures that factories are prepared for peak seasons and can avoid excess inventory during off-peak periods.
-
Cost Efficiency:
-
Minimized Waste: AI-driven re-order optimization reduces waste by ensuring that factories only produce what is needed. This minimization of overproduction leads to cost savings and more sustainable operations.
-
Financial Planning: AI helps factories plan their finances more effectively by providing accurate forecasts and optimizing inventory levels. This financial planning ensures factories can maintain cash flow and invest in growth opportunities.

Implementing AI-driven LATR in Small Garment Factories
AI-Powered Demand Forecasting
-
Tools and Techniques for Accurate Demand Forecasting
-
Machine Learning Algorithms: Machine learning models such as regression analysis, time series forecasting, and neural networks can analyze historical sales data to predict future demand. These models can identify patterns and trends, accounting for seasonality, promotions, and market fluctuations.
-
Real-Time Data Integration: AI tools integrate real-time data from multiple sources, including social media, e-commerce platforms, and market reports. This real-time integration allows for immediate adjustments to forecasts based on current events and emerging trends.
-
Natural Language Processing (NLP): NLP can analyze textual data from customer reviews, social media posts, and online feedback to gauge consumer sentiment and preferences. This qualitative data complements quantitative sales data, providing a more comprehensive demand forecast.
-
Predictive Analytics Platforms: They offer robust predictive analytics tools that enable small garment factories to forecast demand accurately. These platforms use advanced algorithms and data visualization tools to present actionable insights.
2. Case Study of Successful Demand Prediction Using AI
-
EverLighten Success with AI Forecasting
-
Approach: EverLighten implemented machine learning models to analyze historical sales data and integrate real-time data from their e-commerce platform and social media channels. By continuously refining their models, they predicted demand for custom socks and apparel with high accuracy.
-
Results: The AI-driven forecasting reduced stockouts by 25% and excess inventory by 20%, leading to better inventory management and higher customer satisfaction.
Automated Product Testing
-
AI Role in Rapid Prototyping and Testing of New Designs
-
Virtual Design and Simulation: AI-powered design tools can create virtual prototypes of new garment designs. Using 3D modeling and simulation, designers can visualize how different fabrics, patterns, and colors will look and fit without physical samples.
-
AI-Driven Fit Analysis: AI systems can analyze the fit and comfort of new designs by simulating how garments will behave on different body types. This analysis helps identify potential issues early in the design process, reducing the need for physical adjustments.
-
Automated A/B Testing: AI can conduct A/B testing by launching multiple versions of a design to different consumer segments. By analyzing the performance of each version, AI identifies the most appealing design elements and guides future production decisions.
2. Benefits of Automated Testing in Reducing Time-to-Market and Ensuring Product Quality
-
Faster Time-to-Market: AI-driven rapid prototyping and virtual testing significantly reduce the time required to bring new designs to market. Designers can iterate quickly, making adjustments based on AI insights and feedback from virtual simulations.
-
Cost Savings: Automated testing lowers production costs by minimizing the need for physical samples and reducing the number of design iterations. Small garment factories can allocate resources more efficiently, focusing on successful designs.
-
Enhanced Product Quality: Automated testing ensures that new designs meet quality standards before full-scale production. AI's ability to identify potential issues early in the process leads to higher-quality products and fewer defects.
-
Market Responsiveness: Automated testing allows small garment factories to respond to market trends and consumer feedback. By continuously testing and refining designs, factories can stay ahead of fashion trends and maintain a competitive edge.
Efficient Re-Order Management
-
AI Systems for Real-Time Inventory Management and Re-Ordering
-
Real-Time Inventory Tracking: AI-powered inventory management systems provide real-time visibility into stock levels, sales data, and supply chain logistics. This real-time tracking ensures small garment factories have up-to-date information to make informed re-ordering decisions.
-
Automated Re-Ordering Triggers: AI systems can set automated re-ordering triggers based on predefined thresholds. When inventory levels fall below a certain point, AI generates re-orders to replenish stock, ensuring continuous availability of popular items.
-
Supplier Coordination: AI can coordinate with suppliers to ensure timely delivery of materials and components. By optimizing supplier relationships and logistics, AI reduces lead times and maintains smooth production flow.
2. How AI Helps in Maintaining Optimal Stock Levels and Reducing Overproduction
-
Demand-Driven Re-Ordering: AI-driven demand forecasting informs re-ordering decisions, ensuring stock levels align with anticipated demand. The method reduces the likelihood of surplus inventory and overproduction.
-
Safety Stock Optimization: AI can calculate optimal safety stock levels based on historical sales data, demand variability, and lead times. This optimization ensures small garment factories maintain sufficient inventory to meet unexpected demand without overstocking.
-
Inventory Turnover Rate: AI helps improve inventory turnover rates by ensuring that products are re-ordered based on actual sales performance. Higher turnover rates reduce holding costs and minimize the risk of obsolete inventory.
-
Cost Efficiency: By maintaining optimal stock levels and reducing overproduction, AI-driven re-order management enhances cost efficiency. Small garment factories can allocate resources more effectively, focusing on high-demand products and reducing waste.

Case Studies and Success Stories
EverLighten LATR Implementation
Approach: Leveraging AI for Demand Prediction, Automated Testing, and Re-Order Optimization
EverLighten, a small garment factory known for its customized items, adopted the LATR model to stay competitive and responsive to market trends. Here is how they did it:
-
Demand Prediction:
-
AI Tools: EverLighten implemented machine learning algorithms to analyze historical sales data, seasonal trends, and market fluctuations. They used predictive analytics platforms to integrate real-time data from their e-commerce platform and social media.
-
Trend Identification: AI monitored social media platforms to detect emerging trends and consumer preferences. They performed sentiment analysis on customer reviews to gauge consumer sentiment toward different products.
-
Outcome: The AI-driven demand prediction allowed EverLighten to accurately forecast demand for their custom socks and apparel, reducing stockouts by 25% and minimizing excess inventory by 20%.
-
Automated Product Testing:
-
Virtual Prototyping: Using AI-powered design tools, EverLighten created virtual prototypes of new designs. 3D modeling and simulation enabled the design team to visualize how different fabrics, patterns, and colors would look without physical samples.
-
Market Testing: AI managed the production of small batches of new designs for market testing. They analyzed sales data and customer feedback from these batches to determine the success of new products.
-
Outcome: Automated testing reduced the time-to-market for new designs, improved product quality, and ensured the move of successful designs to larger-scale production. This approach led to a 15% reduction in production costs and faster response to market demands.
-
Re-Order Optimization:
-
Real-Time Inventory Management: AI provided real-time visibility into stock levels and sales data. Automated re-ordering triggers were set based on predefined thresholds, ensuring continuous availability of popular items.
-
Supplier Coordination: AI coordinated with suppliers to ensure timely delivery of materials and components. Predictive analytics informed re-order quantities and timing, optimizing stock levels and reducing overproduction.
-
Outcome: The AI-driven re-order optimization enhanced inventory turnover rates, minimized waste, and maintained optimal stock levels. EverLighten achieved a 20% increase in efficiency and a faster response to market trends.
Results:
-
Increased Efficiency: The AI-driven LATR model streamlined operations, reduced manual intervention, and improved overall efficiency.
-
Reduced Waste: Accurate demand prediction and re-order optimization minimized overproduction and excess inventory. It leads to reduced waste.
-
Faster Response to Market Trends: Automated testing and real-time data integration enabled EverLighten to quickly adapt to changing consumer preferences and market demands.

Benefits of AI-Driven LATR for Small Garment Factories
Market Responsiveness
-
Real-Time Data Analysis:
-
Trend Detection: AI systems analyze real-time data from various sources, including social media, e-commerce platforms, and market reports, to detect emerging trends. This immediate trend detection allows factories to adapt their production schedules and introduce new designs that align with consumer preferences.
-
Consumer Feedback Integration: AI can gather and analyze customer feedback from multiple channels, providing valuable insights into consumer sentiments and preferences. By incorporating this feedback into their design and production processes, factories can ensure that their products meet market demands.
-
Agile Production Planning:
-
Dynamic Scheduling: AI-driven production planning systems can adjust production schedules based on real-time demand data. This flexibility allows factories to ramp up production of high-demand items and scale back on less popular products, ensuring they stay responsive to market changes.
-
Inventory Management: AI can optimize inventory levels by predicting demand fluctuations and adjusting stock levels accordingly. This demand-driven inventory management prevents overstocking and stockouts, enabling factories to maintain a steady supply of products in high demand.
-
Quick Decision-Making:
-
Predictive Analytics: AI uses predictive analytics to forecast future demand based on historical data and current market trends. This foresight enables small garment factories to decide which products to prioritize and when to introduce new designs.
-
Automated Re-Ordering: AI systems can automate the re-ordering process, ensuring that factories can quickly replenish stock based on real-time sales data. This automation reduces lead times and ensures that popular products are always available.
Cost Efficiency
-
Optimized Resource Allocation:
-
Efficient Use of Materials: AI can optimize the use of raw materials by predicting the exact amount needed for each production run. This precision reduces waste and lowers material costs. It contributes to overall cost efficiency.
-
Labor Optimization: AI can allocate labor resources based on current production needs, ensuring the utilization of workers effectively. This optimization reduces underutilization and overstaffing, lowering labor costs.
-
Reduced Operational Costs:
-
Inventory Management: AI-driven inventory management systems minimize holding costs by maintaining optimal stock levels. Small garment factories can achieve significant cost savings by preventing overstocking and reducing the risk of obsolete inventory.
-
Predictive Maintenance: AI forecasts machinery maintenance needs by analyzing usage and wear. This proactive approach reduces the risk of unexpected breakdowns and costly repairs, ensuring that machinery operates efficiently.
-
Streamlined Production Processes:
-
Automated Testing: AI-powered testing processes reduce the time and cost of bringing new designs to market. By identifying potential issues early in the design process, factories can avoid costly rework and ensure that only successful designs move to larger-scale production.
-
Demand-Driven Production: AI aligns production schedules with actual market demand, reducing the risk of overproduction and excess inventory. This demand-driven approach ensures efficient use of resources and costs are under control.
Sustainability
-
Minimized Waste:
-
Precise Material Usage: Its ability to predict the exact amount of material needed for each production run minimizes waste. This precision ensures efficient material use, reducing the environmental impact of excess fabric and other raw materials.
-
Optimized Inventory Management: AI prevents overproduction by aligning inventory levels with actual demand. This optimization reduces the amount of unsold inventory that would otherwise go to waste.
-
Energy Efficiency:
-
Smart Energy Management: AI can optimize energy consumption by adjusting machine settings and production schedules to align with energy-efficient practices. This optimization reduces energy costs and contributes to more sustainable operations.
-
Reduced Carbon Footprint: By maintaining optimal stock levels and minimizing waste, AI-driven LATR models reduce the overall carbon footprint of garment factories. This reduction is achieved through efficient use of resources and energy-saving practices.
-
Sustainable Supply Chain Practices:
-
Supplier Collaboration: AI can coordinate with suppliers to ensure timely delivery, reducing the need for expedited shipping and associated carbon emissions. By optimizing supplier relationships, factories can adopt more sustainable supply chain practices.
-
Eco-Friendly Design: AI can assist in designing eco-friendly products by analyzing consumer preferences for sustainable materials and production methods. This analysis enables factories to create products that meet market demands for sustainability.

Conclusion
The Large-Scale Automated Test and Re-Order (LATR) model, enhanced by AI, provides small garment factories with a framework for staying competitive and responsive to market demands. By leveraging AI technologies, factories can accurately predict consumer demand, automate testing of new designs, and optimize re-order quantities and timing. These capabilities improve efficiency, market responsiveness, and sustainability.
Adopting AI-driven LATR models is crucial for small garment factories to achieve sustainable growth and maintain a competitive edge in the fashion industry. The ability to quickly adapt to market changes, optimize production, and reduce waste enhances profitability and aligns with modern sustainability goals. By integrating AI into their operations, small garment factories can unlock new opportunities for innovation and success.
Connect with EverLighten for AI-Driven Solutions:
-
100% Customization: Tailor every aspect of your designs and products to reflect your unique brand identity.
-
100% Quality Check: Rigorous quality assurance processes to ensure the highest standards.
-
Free Design Help: Expert design assistance at no extra cost.
-
Worldwide Delivery: We offer dependable and efficient global shipping.
-
24/7 Support: Around-the-clock support to assist with any inquiries.
-
Unlimited Revisions: We'll work until your design is perfect, with unlimited revisions.
-
Low MOQ (Minimum Order Quantity): Our flexible order quantities support businesses, big and small.
Transform your garment production with us. Contact EverLighten today to explore how our AI-driven solutions can help you reach your business objectives.
FAQs
-
What is the LATR model, and how does it benefit garment factories?
-
The LATR model involves producing small batches of new designs, testing their performance in the market, and quickly re-ordering successful items. It benefits garment factories by reducing waste, improving efficiency, and enhancing market responsiveness.
-
How can AI predict consumer demand for new garment designs?
-
AI uses machine learning algorithms, real-time data integration, and sentiment analysis to forecast consumer demand. AI provides accurate demand predictions by analyzing historical sales data, market trends, and consumer preferences.
-
What are the advantages of using AI for automated product testing in garment factories?
-
AI-driven automated testing speeds up the design and prototyping process reduces costs, and ensures high product quality. By identifying potential issues early, AI allows factories to bring successful designs to market faster.
-
How does AI optimize re-order quantities and timing for small garment factories?
-
AI-driven inventory management systems provide real-time visibility into stock levels and sales data. Automated re-ordering triggers ensure that factories replenish stock based on actual demand, maintaining optimal inventory levels and reducing overproduction.
-
Can you provide examples of successful LATR implementation in garment factories using AI?
-
Yes. EverLighten successfully implemented the LATR model by leveraging AI for demand prediction, automated testing, and re-order optimization. Another small garment factory used AI-driven tools to enhance production flexibility and market responsiveness, achieving significant improvements in efficiency and sustainability.