How AI can enhance the 5 Crucial Steps of Manufacturing in Small Garment Factories and Brands
| Nov, 05 , 24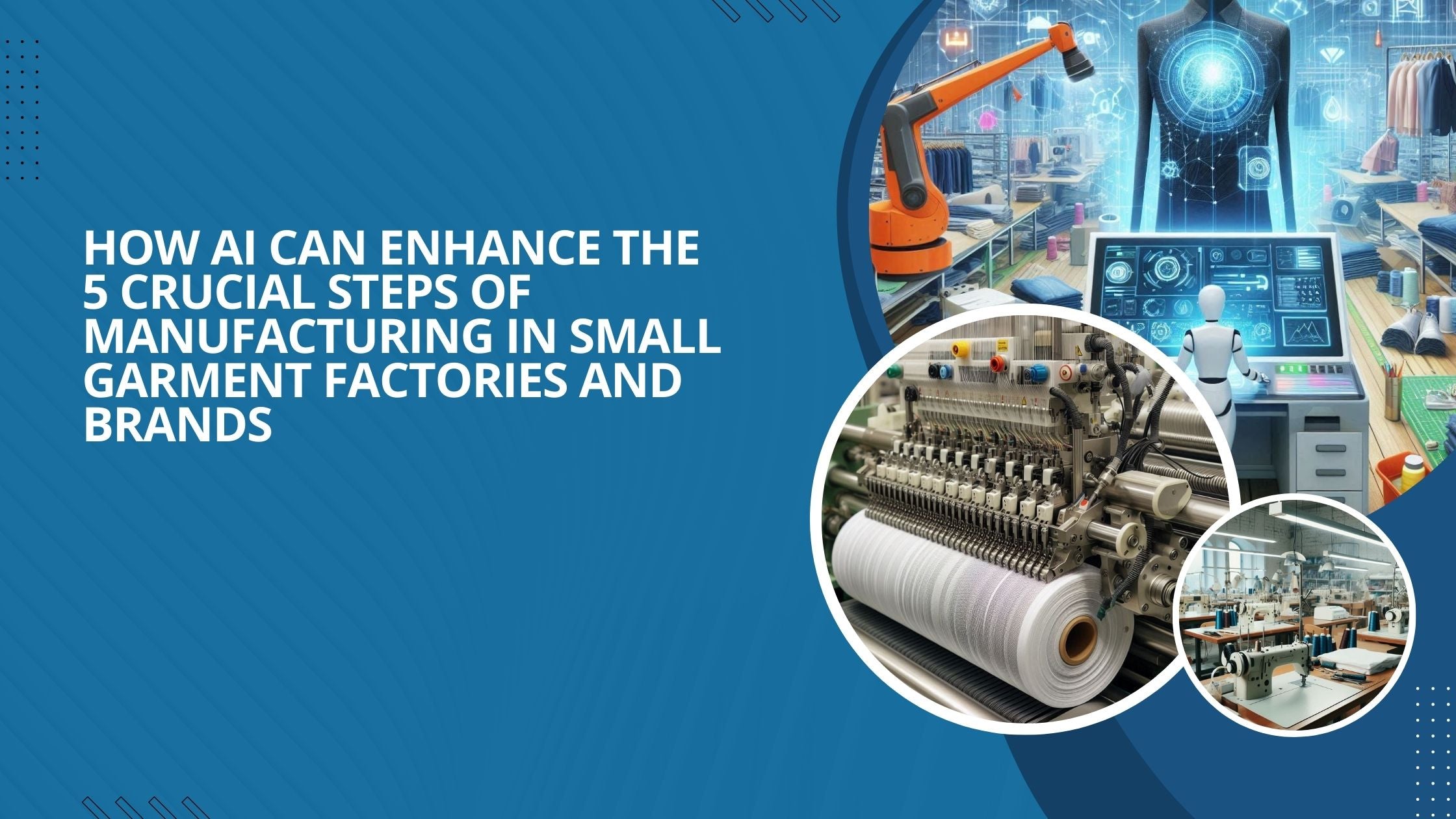
In the fast-paced world of garment manufacturing, staying competitive requires more than creativity and quality—it demands efficiency and precision at every stage of the production process. The challenge is more substantial for small garment factories and brands as they must compete with larger organizations with more resources and technology. The five key stages of garment manufacturing—pre-production, production planning, cutting, manufacturing & quality control, and delivery—are essential to their success but can also be time-consuming and prone to human error.
It is where Artificial Intelligence (AI) is stepping in, transforming the way garments are produced by streamlining processes, reducing waste, and improving accuracy. From material sourcing to faster production planning and more precise cutting techniques, AI is reshaping traditional manufacturing practices and offering a lifeline to small garment brands that need to optimize every step.
In this blog, we will explore these five steps and compare the traditional methods with AI-driven approaches to show how small garment factories and brands can unlock new levels of efficiency and profitability.
Let us begin.

Pre-Production: Materials Sourcing, Pattern Making, and Sampling
Traditional Methods:
- Materials Sourcing: For small garment factories, materials sourcing involves manual processes, from negotiating with suppliers to finding the best quality at the right price. It can be time-consuming and inefficient because it relies heavily on human networks, word-of-mouth referrals, and basic research. In many cases, small factories face the challenge of limited access to a global supply network, which restricts their material choices and pricing options.
- Pattern Making: Traditional pattern making is a labor-intensive process where patterns are drawn by hand or with regular design software. This method can lead to inaccuracies and miscalculations in fit, leading to fabric waste and production errors. For small brands that cannot afford the luxury of multiple revisions, this can result in significant material losses and financial strain.
- Sampling: They produce physical samples to test the look, feel, and fit. While this is critical for ensuring quality, the process can be time-consuming and costly, especially for small garment factories that must create multiple prototypes before arriving at the final design. Physical sampling also adds to production delays and material waste.
AI-Driven Methods:
- AI in Material Sourcing: AI-driven platforms can now analyze massive data sets to optimize material sourcing for small garment factories. Instead of relying on human networks, AI tools can rapidly scan global suppliers based on criteria like pricing, sustainability, quality, and delivery timelines. These platforms offer instant recommendations, allowing small businesses to discover suppliers they might not have known existed, negotiate prices more efficiently, and make more informed decisions.
- Pattern Making: AI-powered pattern-making software generates precise digital patterns that reduce human error and fabric waste. These tools produce more accurate patterns by using AI to analyze measurements, garment structure, and fit. Small brands benefit from minimized material costs and a reduced need for multiple pattern revisions, allowing them to create high-quality garments with greater precision.
- Digital Sampling: AI has made virtual sampling a reality. Instead of producing physical prototypes, AI-driven tools allow digital samples to be reviewed and adjusted in real time. It significantly shortens lead times, as small garment factories no longer need to wait for physical samples to be shipped, evaluated, and revised. Virtual samples allow for quicker decision-making and cost savings in materials and labor.
Key Benefits of AI in Pre-Production:
- Faster and More Cost-Efficient Processes: AI accelerates material sourcing, pattern making, and sampling, helping small brands compete in a fast-moving industry.
- Reduced Wastage and Higher Precision: AI improves pattern-making accuracy, reducing fabric waste and ensuring better garment fit.
- Quicker Approvals Through Virtual Sampling: Digital samples streamline the approval process, cutting production timelines and costs.

Production Planning: Forecasting and Scheduling
Traditional Methods:
- Forecasting: Small garment factories often rely on historical data and human intuition to forecast demand. While this method can work, it is prone to inaccuracies, especially in a fast-changing market. Poor demand forecasting can lead to underproduction or overproduction. It can hurt profitability. This manual forecasting method can not predict sudden market shifts or emerging trends.
- Scheduling: Production planning is typically a reactive process, with human planners managing the entire schedule based on past experiences. Small garment factories often face bottlenecks and inefficiencies due to unforeseen disruptions, such as delays in material delivery or unexpected surges in demand. It can lead to missed deadlines and rushed production runs. It can compromise quality and increase stress on the workforce.
AI-Driven Methods:
- AI in Forecasting: AI excels in analyzing real-time data, market trends, and consumer behavior, making demand forecasting far more accurate. By leveraging machine learning algorithms, AI tools can predict future trends, demand surges, and consumer preferences. It enables small garment factories to adjust production volumes, avoiding the costs associated with overproduction or stockouts.
- Optimized Scheduling: AI-powered tools can automate and optimize production schedules by analyzing data points like labor availability, material supply, and machine capacity. AI algorithms can dynamically adjust schedules in response to real-time market changes, ensuring smoother operations and fewer disruptions. It can be a game changer for small garment factories and brands because it allows them to avoid bottlenecks, make faster decisions, and improve overall production efficiency.
Key Benefits of AI in Production Planning:
- Improved Forecasting Accuracy: AI helps small factories predict market demand with higher precision, reducing the risks of excess inventory or stock shortages.
- Dynamic Scheduling: AI enables small garment factories to adapt in real-time to shifts in demand, supplier delays, or workforce availability, enhancing production flexibility and reducing downtime.

Cutting Process: Improving Precision and Reducing Waste
Traditional Methods:
- Manual Cutting: Traditionally, cutting fabric in small garment factories is manual. Workers use scissors or cutting tools to follow patterns, but this approach is prone to errors and imprecision. Inaccurate cutting often results in substantial fabric wastage, which is costly for smaller brands that operate on tight margins.
- Laser-Cutting Technology: While more advanced than manual methods, laser-cutting technology still requires manual setting and control. Without automated adjustments, the risk of human error remains high. If the settings are slightly off, it can lead to inaccurate cuts, wasted materials, and inconsistent garment production.
AI-Driven Methods:
- AI-Guided Cutting: AI-driven cutting technology improves fabric utilization. By analyzing pattern data, AI can determine the most efficient way to position patterns on fabric, ensuring efficient use of every inch. It minimizes waste and allows small factories to maximize their fabric supplies, which is particularly important when working with expensive or limited materials.
- Self-Adjusting Systems: AI-integrated cutting machines can automatically adjust their settings based on fabric type, thickness, and specific design requirements. These systems can fine-tune their operations in real-time, eliminating the need for manual adjustments and reducing the chance of errors. It is helpful when working with complex or delicate fabrics that require precise handling.
Key Benefits of AI in the Cutting Process:
- Reduced Fabric Waste: AI optimizes fabric layout, drastically reducing material waste, which is especially critical for small brands trying to maintain profitability.
- Increased Accuracy and Efficiency: AI improves precision in cutting, even with intricate designs, leading to fewer errors, faster production times, and consistent garment quality.

Manufacturing and Quality Control: Ensuring Consistency and Speed
Traditional Methods:
- Labor-Intensive Manufacturing: They rely heavily on manual labor. Workers perform tasks such as sewing, stitching, and assembly, which can lead to inconsistencies in quality. Fatigue, human error, and varying skill levels among workers can result in uneven stitching, misaligned seams, or other production flaws.
- Manual Quality Control: Quality control in traditional manufacturing involves human inspection, where teams visually examine each product for defects. While effective to some degree, this process is time-consuming and subject to human error. Minor flaws can go unnoticed, and the manual inspection process can cause production delays, affecting delivery schedules.
AI-Driven Methods:
- Automated Manufacturing: AI-powered robots and machines can automate repetitive and precise tasks like sewing and stitching. These machines maintain consistent quality throughout production, significantly reducing the risk of human error. AI ensures that every garment is produced to the same high standard, regardless of the complexity of the design.
- AI in Quality Control: AI-driven quality control systems use image recognition, machine learning, and data analysis to detect even the tiniest defects. These systems can analyze a product in real-time, instantly flagging issues such as uneven stitching, misalignment, or fabric imperfections. It ensures that only flawless garments proceed to the next production stage, eliminating the need for rework and reducing material waste.
Key Benefits of AI in Manufacturing and Quality Control:
- Faster and More Consistent Manufacturing: AI increases production speed by automating labor-intensive tasks while maintaining consistency across all garments.
- Real-Time Defect Detection: AI-enabled quality control ensures early detection of errors. It reduces rework and waste. It refines the final product's quality and appearance.

Delivery: Streamlining the Supply Chain
Traditional Methods:
- Manual Logistics Planning: Delivery logistics are often managed manually for small garment factories. It involves coordinating with shipping companies, handling customs paperwork, and planning delivery routes based on human judgment. Manual planning is prone to inefficiencies, including delivery delays. It can become more challenging because of unforeseen circumstances such as weather disruptions or supply chain bottlenecks.
- Limited Visibility in the Supply Chain: Traditional logistics systems often lack full transparency, making it difficult for small factories to track shipments in real-time. It can result in delayed responses to problems such as missing or late shipments, causing frustration and affecting the reputation.
AI-Driven Methods:
- AI in Logistics: AI-powered logistics systems offer advanced tools for optimizing delivery routes, managing shipping schedules, and predicting supply chain disruptions. AI algorithms can analyze real-time data to identify potential bottlenecks, such as traffic delays or port congestion, and adjust delivery plans accordingly. It ensures faster and more efficient distribution of products, allowing small brands to compete with larger companies with more established supply chains.
- Real-Time Tracking: AI-enabled tracking systems provide end-to-end visibility for manufacturers and customers. With real-time data on shipments, manufacturers can monitor the progress of deliveries and make adjustments as needed. Customers also benefit from the ability to track their orders, leading to increased trust and satisfaction.
Key Benefits of AI in Delivery:
- Optimized Supply Chain Management: AI predictive capabilities help prevent delays and optimize delivery routes, ensuring products reach customers on time.
- Increased Customer Satisfaction with real-time tracking provides complete transparency. It improves communication and their overall experience.

Conclusion
AI is revolutionizing garment manufacturing at every stage, from pre-production to delivery. Small garment factories often face efficiency, material costs, and quality control challenges that can benefit from AI-driven solutions. By automating vital tasks, reducing waste, improving precision, and streamlining the supply chain, AI enables small brands to compete more effectively with larger companies.
Small garment factories that embrace AI technology will see improvements in production speed, cost-efficiency, and customer satisfaction, ultimately increasing profitability. Adopting AI-driven processes will be vital for staying competitive.
If you're a brand looking to enhance your garments, connect with EverLighten. We offer tailored solutions to help you optimize your production, reduce waste, and deliver high-quality products to your customers.
FAQs:
How can AI reduce fabric waste during the cutting process?
AI optimizes fabric layout by analyzing pattern data and determining the most efficient cutting patterns. It leads to minimal fabric waste.
Can AI improve the quality of garments produced in small factories?
Yes, AI-driven quality control systems provide real-time defect detection, ensuring that every garment meets high standards.
What are the benefits of AI in production planning?
AI enhances forecasting and scheduling accuracy by using real-time data and market trends, allowing small factories to adapt quickly to changes.
How does AI impact delivery logistics in small factories?
AI tools optimize delivery routes and predict potential supply chain bottlenecks, ensuring faster and more reliable shipments.
Is AI affordable for small garment factories?
Many AI-driven solutions are scalable and affordable. It makes them accessible to small brands looking to improve manufacturing efficiency and reduce costs.